Pseudo R Squared as a Measure of Fit
The Pseudo R Squared Problem
When searching in 1988 for an R squared analogue for use with a probit equation, a review of the econometrics literature led to about a dozen proposed formulas, but no clear indication of the absolute or even relative merits of any of them. It was just a list. Some of the proposals had real problems. For example, in a linear model, R squared takes on a value of zero when there is no explanatory power and a value of 1 when the fit is perfect. The zero was not a problem for the proposed analogues, but some of the measures based on the likelihood function were bounded above by values substantially less than 1.
Other likelihood-based measures achieved the unit upper bound at the cost of multiplying by whatever factor assures convergence to 1 for a perfect fit. These included the frequently encountered McFadden pseudo R squared, for which the multipicative adjustment factor can be greater than or less than 1, depending on the data. The problem with these formulas is that the ad hoc multiplicative factor makes them increase too quickly or too slowly as the fit of the model improves from zero. For example, the McFadden measure overstates the fit of the model if one of the two values of the dependent variable occurs less than 19.97% of the time in the sample, and it otherwise understates the fit.
Other alternatives were based on second moments of the actual or fitted values of the variables in the equation, mimicking formulas that work in the linear model. These measures are consistent with the likelihood function of the linear model, which is closely related to second moments, but are not consistent with the likelihood function for dichotomous dependent variable models, which is logarithmic to avoid problems with heteroskedasticity.
Solution
The solution to the problems of the existing measures was conceptually clear: construct a likelihood-based measure that covers the right range of values and grows with the fit of the model at a pace analogous to that of the linear model. In that case, its interpretation would be similar to that of the linear model R squared. The solution is derived in the excerpt below from Estrella (1998) by solving a differential equation that gives precise mathematical meaning to the conceptual goal.

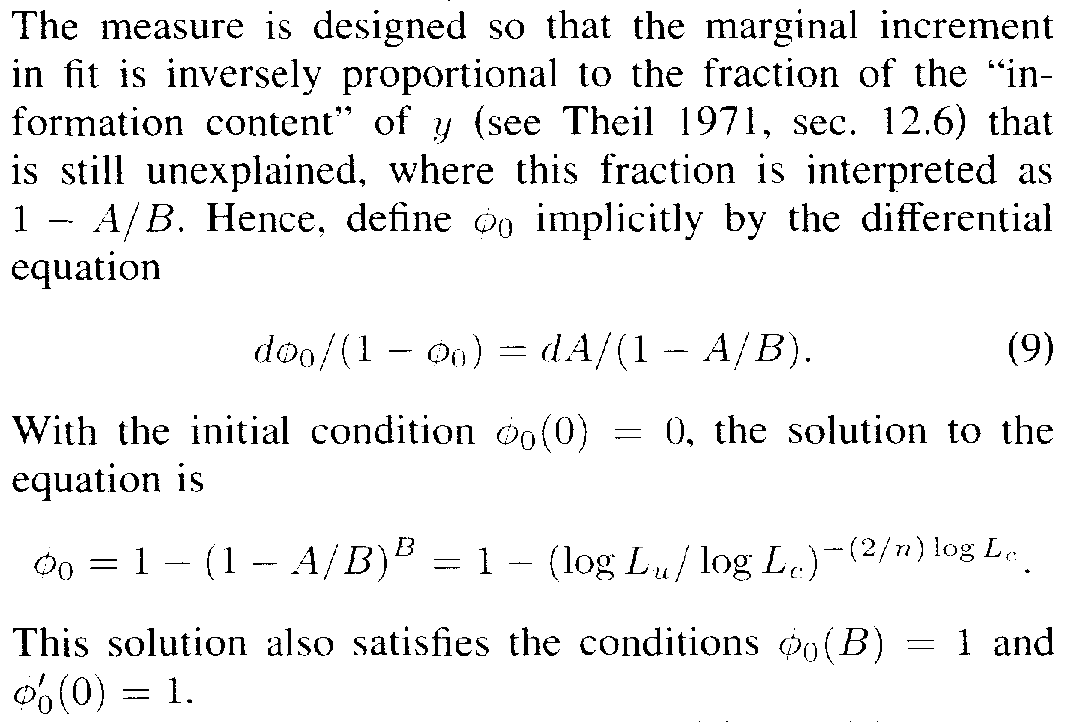
This R squared has applications to other limited dependent variable models beyond the dichotomous case. It may also be adjusted for the number of estimated parameters in ways analogous to AIC and BIC and to adjusted R squared in the linear model.
Development
Estrella, Arturo (1998) “A new measure of fit for equations with dichotomous dependent variables.” Journal of Business and Economic Statistics. Working Paper.
Illustrative Applications
Estrella, Arturo and Frederic S. Mishkin (1997) “The predictive power of the term structure of interest rates in Europe and the UnitedStates: Implications for the European central bank.” European Economic Review, July 1997
Bernard, Henri and Stefan Gerlach (1998) “Does the term structure predict recessions? The international evidence.” International Journal of Finance and Economics. Working Paper.
Estrella, Arturo and Frederic S. Mishkin (1998) “Predicting U.S. recessions: Financial variables as leading indicators.” Review of Economics and Statistics.
Estrella, Arturo, Stavros Peristiani and Sangkyun Park (2000) “Capital ratios as predictors of bank failures.” Federal Reserve Bank of New York Economic Policy Review.
Davier, Frédéric F. (2001) “L'importance de la pénétration des technologies de l'information et de la communication en Suisse.” Swiss Journal of Economics and Statistics.
Estrella, Arturo, Stavros Peristiani and Sangkyun Park (2002) “Capital ratios and credit ratings as predictors of bank failures.” In Ong, Michael K., ed., Credit Ratings: Methodologies, Rationale and Default Risk, London: Risk Books.
Estrella, Arturo, Anthony P. Rodrigues and Sebastian Schich (2003) “How stable is the predictive power of the yield curve? Evidence from Germany andthe United States.” Review of Economics and Statistics.
Moneta, Fabio (2005) “Does the yield spread predict recessions in the Euro Area?.” International Finance.
Tobias Adrian and Arturo Estrella (2008) "Monetary Tightening Cycles and the Predictability of Economic Activity." Economics Letters.
Nyberg, Henri (2012) “Risk-return tradeoff in U.S. stock returns over the business cycle.” Journal of Financial and Quantitative Analysis.
Christiansen, Charlotte and Jonas Eriksen (2014) "Forecasting US recessions: The role of sentiment." Journal of Banking & Finance
Pönkä, Harri and Markku Stenborg (2020) “Forecasting the state of the Finnish business cycle.” Finnish Economic Papers.
Stuart, Rebecca (2020) “Monetary regimes, the term structure and business cycles in Ireland, 1972–2018.” Manchester School.